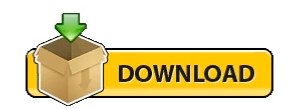
You get the best of speed and flexibility for your crazy research. While this technique is not unique to PyTorch, it's one of the fastest implementations of it to date. Our inspiration comesįrom several research papers on this topic, as well as current and past work such as With PyTorch, we use a technique called reverse-mode auto-differentiation, which allows you toĬhange the way your network behaves arbitrarily with zero lag or overhead.
One has to build a neural network and reuse the same structure again and again.Ĭhanging the way the network behaves means that one has to start from scratch.
Most frameworks such as TensorFlow, Theano, Caffe, and CNTK have a static view of the world. PyTorch has a unique way of building neural networks: using and replaying a tape recorder. Such as slicing, indexing, math operations, linear algebra, reductions.Īnd they are fast! Dynamic Neural Networks: Tape-Based Autograd We provide a wide variety of tensor routines to accelerate and fit your scientific computation needs PyTorch provides Tensors that can live either on the CPU or the GPU and accelerates the If you use NumPy, then you have used Tensors (a.k.a.
A deep learning research platform that provides maximum flexibility and speed.Įlaborating Further: A GPU-Ready Tensor Library. A replacement for NumPy to use the power of GPUs. Useful for data loading and Hogwild trainingĭataLoader and other utility functions for convenience Python multiprocessing, but with magical memory sharing of torch Tensors across processes. More About PyTorchĪt a granular level, PyTorch is a library that consists of the following components: ComponentĪ Tensor library like NumPy, with strong GPU supportĪ tape-based automatic differentiation library that supports all differentiable Tensor operations in torchĪ compilation stack (TorchScript) to create serializable and optimizable models from PyTorch codeĪ neural networks library deeply integrated with autograd designed for maximum flexibility Dynamic Neural Networks: Tape-Based Autograd. You can reuse your favorite Python packages such as NumPy, SciPy, and Cython to extend PyTorch when needed. Deep neural networks built on a tape-based autograd system. Tensor computation (like NumPy) with strong GPU acceleration. PyTorch is a Python package that provides two high-level features: